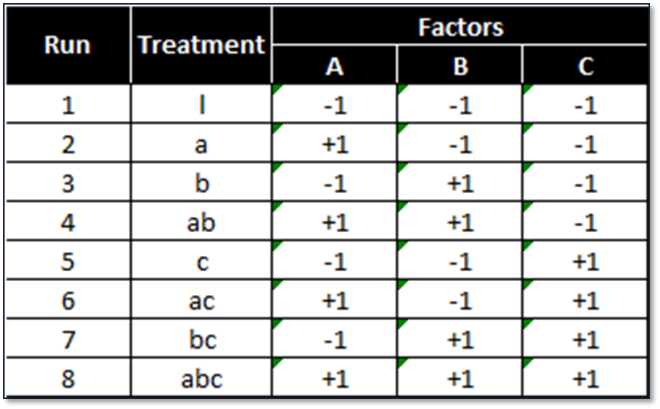
The Main Total Effect can be related to input variables by moving along the row and looking at the first column. If the row in the first column is a2b1c1 then the main total effect is A. It is clear that in order to find the total factorial effects, you would have to find the main effects of the variable and then the coefficients.
Pandas: How to Sort DataFrame Based on String Column
People who were rewarded spotted a few more differences than the people who weren’t, but this doesn’t tell us if they were any less distracted. We will be imagining experiments that are trying to improve students grades. So, the dependent variable will always be grade on a test.
2 Designs
So, our measure of performance, our dependent variable, could be the mean number of differences spotted. Before we go on, let’s clarify what we mean by manipulating more than one thing at once. When you have one IV in your design, by definition, you are manipulating only one thing. This might seem confusing at first, because the IV has more than one level, so it seems to have more than one manipulation. Consider manipulating the number of coffees that people drink before they do a test.
How to Rename the Rows in a Pandas DataFrame
In our example above we showed you two bar graphs of the very same means for our 2x2 design. Even though the graphs plot identical means, they look different, so they are more or less easy to interpret by looking at them. Results from 2x2 designs are also often plotted with line graphs. There are four different graphs in Figure 9.7, using bars and lines to plot the very same means from before. We are showing you this so that you realize how you graph your data matters because it makes it more or less easy for people to understand the results. Also, how the data is plotted matters for what you need to look at to interpret the results.
Main Effects
Or, we might want to know what makes people worse at ignoring things. In other words we want to find out what manipulations control the size of the distraction effect (make it bigger or smaller, or even flip around!). For the first level of Time of Day (morning), we measure test performance when some people drank caffeine and some did not. So, in the morning we manipulate whether or not caffeine is taken. Also, in the second level of the Time of Day (afternoon), we also manipulate caffeine. Some people drink or don’t drink caffeine in the afternoon as well, and we collect measures of test performance in both conditions.
Some examples of bar and line graphs are presented in the margin, and two example tables are presented below. We can do the very same thing to find the main effect of hats. Except in this case, we find the average heights in the no hat vs. hat conditions by averaging over the shoe variable. As expected, we see that the average height is 1 inch taller when subjects wear shoes vs. do not wear shoes.
How to Read the Chi-Square Distribution Table
When you have an interaction effect it is impossible to describe your results accurately without mentioning both factors. You can always spot an interaction in the graphs because when there are lines that are not parallel an interaction is present. If you observe the main effect graphs above, you will notice that all of the lines within a graph are parallel. In contrast, for interaction effect graphs, you will see that the lines are not parallel. The second way of looking at the interaction is to start by looking at the other variable. For example, does the effect of time since last meal depend on the levels of the tired variable?
Instead, if we have done the calculations of the \(SS\)es correctly, they should be same as what we would get if we used R to calculate the \(SS\)es. Let’s make R do the work, and then compare to check our work. Even though this textbook meant to explain things in a step by step way, we guess you are tired from watching us work out the 2x2 ANOVA by hand. We have already shown you how to compute the SS for error before, so we will not do the full example here. Instead, we solve for SS Error using the numbers we have already obtained.
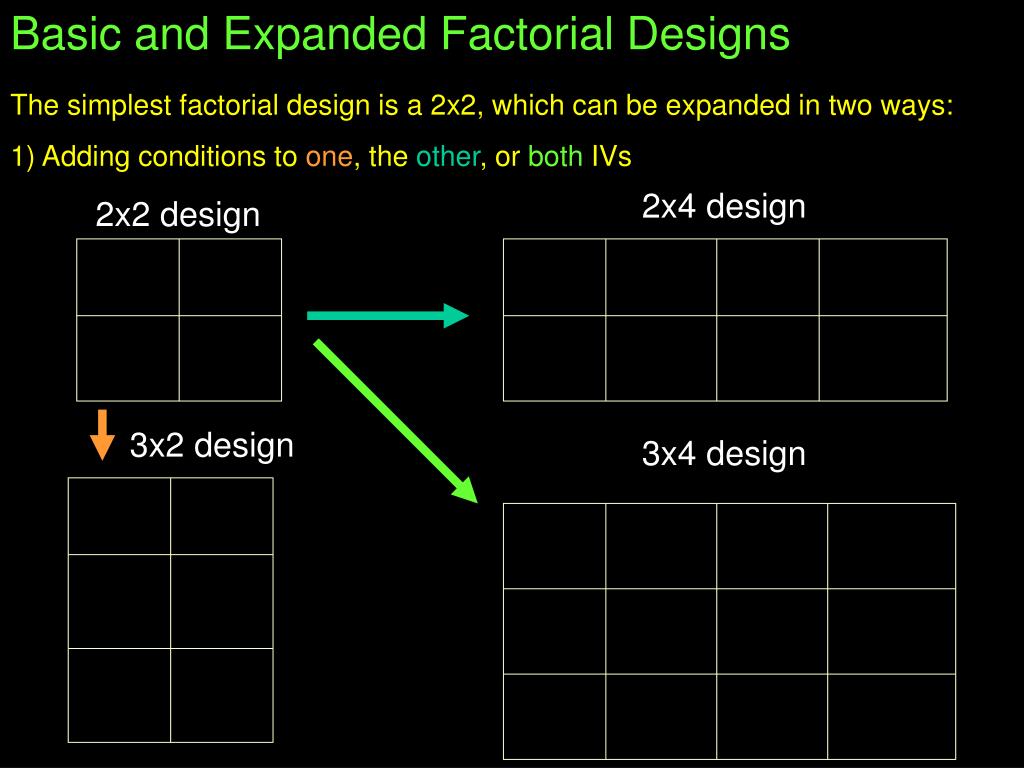
1.1: Factorial Notations and Square Tables
HOPE-3 wades into fray regarding optimal blood pressure targets - MDedge
HOPE-3 wades into fray regarding optimal blood pressure targets.
Posted: Sun, 08 Oct 2017 07:00:00 GMT [source]
Just looking at the means, we can see there was a main effect of reward. The mean number of differences spotted was 11.3 in the reward condition, and 6.6 in the no-reward condition. The Pareto charts show which factors have statistically significant effects on the responses. As seen in the above plots, RPM has significant effects for both responses and pressure has a statistically significant effect on wt% methanol in biodiesel. Neither flow rate or ratio have statistically significant effects on either response.
If we made a separate graph for the main effect of shoes we should see a difference of 1 inch between conditions. Similarly, if we made a separate graph for the main effect of hats then we should see a difference of 6 between conditions. Perhaps the most clear is the main effect of wearing a hat. The red bars show the conditions where people wear hats, and the green bars show the conditions where people do not wear hats. For both levels of the wearing shoes variable, the red bars are higher than the green bars.
Again, you could have put Department as IV1 (columns) and Intervention as IV2 (rows). And again, participants in each “cell” of this design have a unique combination of IV conditions. In addition to the above effects plots, Minitab calculates the coefficients and constants for response equations. The response equations can be used as models for predicting responses at different operating conditions (factors). The coefficients and constants for wt% methanol in biodiesel and number of theoretical stages are shown below.
So by looking at how many numbers are in the notation, you can determine how many independent variables there are in the experiment. 2 x 2, 3 x 3, and 2 x 3 designs all have two numbers in the notation and therefore all have two independent variables. The numerical value of each of the numbers represents the number of levels of each independent variable. A 2 means that the independent variable has two levels, a 3 means that the independent variable has three levels, a 4 means it has four levels, etc.
SOLVE-TAVI: Self-Expandable vs. Balloon-Expandable Valves Yield Similar Outcomes - DocWire News
SOLVE-TAVI: Self-Expandable vs. Balloon-Expandable Valves Yield Similar Outcomes.
Posted: Mon, 05 Jun 2023 12:05:46 GMT [source]
In the distraction condition, there are means for spot-the-difference performance in the no-reward (red), and reward (aqua) conditions. The same goes for the no-distraction condition, a red and an aqua bar for the no-reward and reward conditions. We can try to interpret this graph, but Figure 9.6 plots the same data in a way that makes it easier to see what we are talking about. This is the difference between the AB column (average of subject scores in the no-reward condition) and the CD column (average of the subject scores in the reward condition). These differences for each subjecct are shown in the last green column.
You could run a paired samples \(t\)-test between the mean no-distraction scores for each subject (column AC) and the mean distraction scores for each subject (column BD). Or, you could run a one-sample \(t\)-test on the difference scores column, testing against a mean difference of 0. Maybe rewarding people with money can help you pay attention and ignore distracting things better. We could give people 30 seconds to find as many differences as they can. Then we give them another set of pictures and do it again. Every time we will measure how many differences they can spot.
No comments:
Post a Comment